David Gamarnik lecture - CANCELLED
- 11 November 2020
- Mendel Museum´s Augustinian Abbey Refectory at Mendel Square
We are sorry to announce that this lecture is cancelled. Please follow our website for updates.
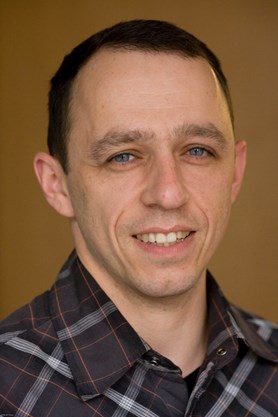
David Gamarnik is the Nanyang Technological University Professor of Operations Research at the MIT Sloan School of Management. His research interests involve probability, stochastic processes, queueing theory, random graphs and probabilistic analysis of combinatorial structures, algorithms and combinatorial optimization, statistics and learning theory. He served as a research staff member at the Department of Mathematical Sciences, IBM Research, where he worked on various projects with industrial applications, including disaster recovery, performance in business processes, call centers, and operational resilience. His research work has been recognized by awards of the 2004 Erlang Prize and the 2011 Best Publication Award from the INFORMS Applied Probability Society.
Abstract
Many combinatorial optimization problems defined on random instances, such as random graphs, exhibit an apparent gap between the optimal values, which can be computed by non-constructive means, and the best values achievable by fast (polynomial time) algorithms. Through a combined effort of mathematicians, computer scientists and statistical physicists, it became apparent that a potential, and in some cases, a provable barrier for designing fast algorithms bridging this gap is an intricate topology of nearly optimal solutions, in particular the presence of a certain Overlap Gap Property (OGP), which we will introduce in this talk. We will demonstrate how for many such problems the onset of the OGP phase transition indeed nearly coincides with algorithmically hard regimes and provides a provable barrier to a broad class of polynomial time algorithms. Our examples will include the problem of finding a largest independent set of a random graph, finding a largest cut in a random hypergrah, random NAE-K-SAT problem, the problem of finding a largest submatrix of a random matrix, the problem of finding a ground state of a p-spin model, and also many models in high-dimensional statistics and machine learning fields.
Share event